Several potential barriers must be addressed as AI algorithms are integrated into diagnostic teams. For example, liability in the event of a missed, inaccurate, or delayed diagnosis is an active area of discussion.44 In addition, payer reimbursement strategies for the use of AI algorithms in the diagnostic process are evolving. Finally, attention must be given to the potential for historically marginalized individuals to be negatively impacted by biases perpetuated by algorithms and unequal access to effective algorithms. Addressing these challenges will require engagement from all members of the diagnostic ecosystem (Figure 3).
National Healthcare Quality and Disparities Report
Latest available findings on quality of and access to health care
Data & Analytics
- Data Infographics
- Data Visualizations
- Data Tools
- Data Innovations
- All-Payer Claims Database
- Consumer Assessment of Healthcare Providers and Systems (CAHPS®) Program
- Healthcare Cost and Utilization Project (HCUP)
- Medical Expenditure Panel Survey (MEPS)
- National Healthcare Quality and Disparities Report Data Tools
- Network of Patient Safety Databases
- AHRQ Quality Indicator Tools for Data Analytics
- Surveys on Patient Safety Culture
- United States Health Information Knowledgebase (USHIK)
- Data Sources Available from AHRQ
Reimagining Healthcare Teams: Leveraging the Patient-Clinician-AI Triad To Improve Diagnostic Safety
Barriers
Table of Contents
- Reimagining Healthcare Teams: Leveraging the Patient-Clinician-AI Triad To Improve Diagnostic Safety
- Introduction
- The Patient-Clinician Dyad
- The Patient-Artificial Intelligence Dyad
- The Clinician-Artificial Intelligence Dyad
- The Patient-Clinician-Artificial Intelligence Triad
- Core Principles for the PCA Diagnostic Team
- Barriers
- Conclusion
- References
Page last reviewed July 2023
Page originally created July 2023
Internet Citation: Barriers. Content last reviewed July 2023. Agency for Healthcare Research and Quality, Rockville, MD.
https://www.ahrq.gov/diagnostic-safety/resources/issue-briefs/dxsafety-reimagining-healthcare-teams-7.html
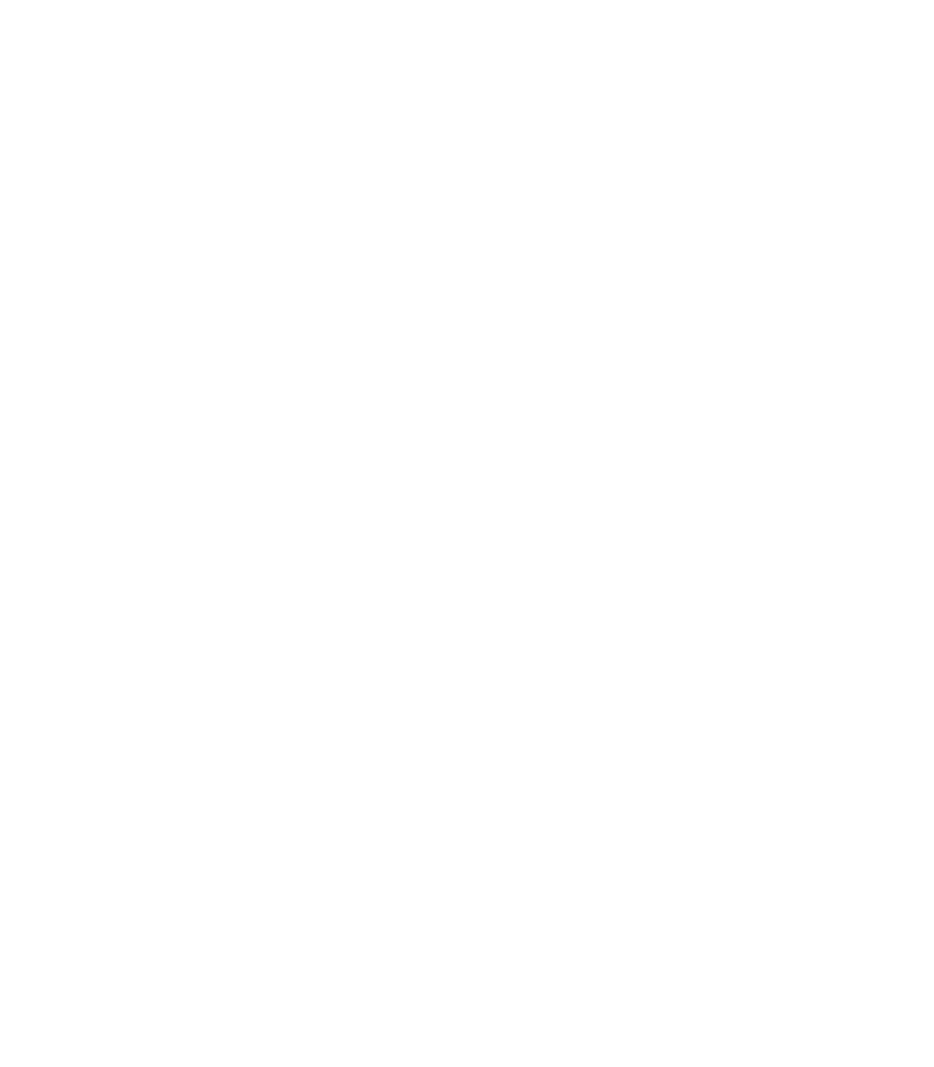